Hi Jason,
This sounds like an interesting problem! Since you are attempting to discern between beeps, you’ve correctly identified that the model needs to be aware of the gaps between the beeps, and how long they are.
The default audio model in Edge Impulse uses 1D convolutions with a relatively small kernel size, which means that it is mostly sensitive to the frequency distribution of sounds within each short slice of audio (as configured in the “frame length” setting of the MFCC block, which is 0.2 seconds by default), but not so much to the way that the audio changes over time. This works great if it’s the “texture” of the audio we care about (for example, discerning between background noise and the sound of running water), but it won’t work as well if timing is important. To incorporate more timing information, we can use 2D convolutions, which look across both axes of the data.
I’m actually working on adding 2D convolutions to the model builder UI right now, but until that’s ready you could try copying and pasting this model into the NN block’s expert view. To open the expert view, click this button:
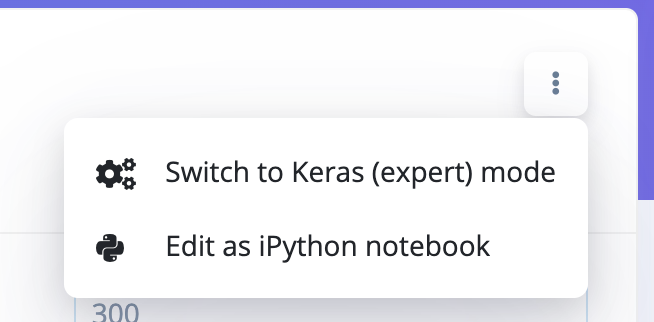
You can then paste in the following model:
import tensorflow as tf
from tensorflow.keras.models import Sequential
from tensorflow.keras.layers import Dense, InputLayer, Dropout, Conv1D, Flatten, Reshape, MaxPooling1D, BatchNormalization, Conv2D, MaxPooling2D, AveragePooling2D
from tensorflow.keras.optimizers import Adam
from tensorflow.keras.constraints import MaxNorm
# model architecture
model = Sequential()
model.add(InputLayer(input_shape=(X_train.shape[1], ), name='x_input'))
model.add(Reshape((int(X_train.shape[1] / 13), 13, 1), input_shape=(X_train.shape[1], )))
model.add(Conv2D(10, kernel_size=5, activation='relu', padding='same', kernel_constraint=MaxNorm(3)))
model.add(AveragePooling2D(pool_size=2, padding='same'))
model.add(Conv2D(5, kernel_size=5, activation='relu', padding='same', kernel_constraint=MaxNorm(3)))
model.add(AveragePooling2D(pool_size=2, padding='same'))
model.add(Flatten())
model.add(Dense(classes, activation='softmax', name='y_pred', kernel_constraint=MaxNorm(3)))
# this controls the learning rate
opt = Adam(lr=0.005, beta_1=0.9, beta_2=0.999)
# train the neural network
model.compile(loss='categorical_crossentropy', optimizer=opt, metrics=['accuracy'])
model.fit(X_train, Y_train, batch_size=32, epochs=9, validation_data=(X_test, Y_test), verbose=2)
To find the smallest model that works, you can experiment with the number of layers, the number of filters and kernel size for each layer. A network based around 2D convolutions will be a lot larger than the equivalent 1D convolutional network, which is why we use the 1D by default.
You should also adjust the number of training epochs until you see that the model’s validation accuracy is no longer improving.
As I mentioned, we’re making improvements this week that will allow you to build these types of models without having to use the advanced view. But in the mean time, let me know how you get on with this model!
Warmly,
Dan